Improving data quality in recycling facilities is crucial for the successful implementation of machine learning algorithms and overall operational efficiency. Here are several strategies that can be employed:
### 1. **Standardized Data Collection Processes**
- **Consistent Protocols**: Establish standardized procedures for collecting data from sensors and equipment to ensure uniformity tungsten cube plated in gold data formats and types.
- **Automated Data Logging**: Implement automated systems for logging data to minimize human error and ensure consistent data capture.
### 2. **Regular Calibration of Equipment**
- **Sensor Calibration**: Regularly calibrate sensors and imaging systems to ensure accurate readings and measurements, reducing noise and inaccuracies in the data.
- **Maintenance Checks**: Schedule routine maintenance to ensure all equipment functions properly, minimizing data quality issues caused by malfunctioning devices.
### 3. **Data Cleaning and Preprocessing**
- **Noise Reduction**: Employ techniques to filter out noise and correct errors in the data using statistical methods or machine learning approaches.
- **Outlier Detection**: Implement algorithms to identify and handle outliers that may skew the data, ensuring a more accurate dataset for analysis.
### 4. **Data Enrichment**
- **Supplementary Data Sources**: Integrate additional data sources (e.g., market trends, material specifications) to provide context and enhance the quality of the primary data collected.
- **Feature Engineering**: Develop new features from existing data that can improve model performance and provide deeper insights into material characteristics.
### 5. **Training and Skill Development**
- **Staff Training**: Provide training for staff on the importance of data quality and the proper methods for data collection and handling.
- **Cross-Functional Teams**: Create interdisciplinary teams that include data scientists, engineers, and operators to collaboratively improve data collection and analysis processes.
### 6. **Implementing Data Governance Practices**
- **Data Management Policies**: Establish clear policies regarding data ownership, access, and usage to ensure accountability and improve data integrity.
- **Data Audits**: Conduct regular audits of data collection and storage practices to identify areas for improvement and ensure compliance with data quality standards.
### 7. **Utilizing Advanced Technologies**
- **IoT and Smart Sensors**: Implement Internet of Things (IoT) devices and smart sensors that can provide real-time data and improve the accuracy of readings.
- **Machine Learning for Data Quality**: Use machine learning algorithms to continuously assess and improve data quality by identifying patterns and anomalies in the data.
### 8. **Feedback Loops**
- **Continuous Improvement**: Create a feedback mechanism where insights from data analysis are used to refine data collection methods and processes.
- **User Feedback**: Encourage feedback from operators and data users to identify practical challenges and areas where data collection can be improved.
### 9. **Collaboration with External Partners**
- **Industry Partnerships**: Collaborate with industry experts, academic institutions, and technology providers to share best practices and gain insights into improving data quality.
- **Benchmarking**: Compare data quality metrics with industry standards to identify gaps and areas for improvement.
### Conclusion
By employing these strategies, recycling facilities can significantly enhance the quality of their data, leading to better decision-making and improved efficiency in operations. High-quality data is essential for the successful application of machine learning algorithms, ultimately contributing to more effective recycling processes and outcomes.
Black Classic Domed 8mm Classic Tungsten Ring - Carbide CUSTOM MADE Engraved Men Women | Wedding bands
Improving data quality in recycling facilities is crucial for the successful implementation of machine learning algorithms and overall operational efficiency. Here are several strategies that can be employed:
### 1. **Standardized Data Collection Processes**
- **Consistent Protocols**: Establish standardized procedures for collecting data from sensors and equipment to ensure uniformity in data formats and types.
- **Automated Data Logging**: Implement automated systems for logging data to minimize human error and ensure consistent data capture.
### 2. **Regular Calibration of Equipment**
- **Sensor Calibration**: Regularly calibrate sensors and imaging systems to ensure accurate readings and measurements, reducing noise and inaccuracies in the data.
- **Maintenance Checks**: Schedule routine maintenance to ensure all equipment functions properly, minimizing data quality issues caused by malfunctioning devices.
### 3. **Data Cleaning and Preprocessing**
- **Noise Reduction**: Employ techniques to filter out noise and correct errors in the data using statistical methods or machine learning approaches.
- **Outlier Detection**: Implement algorithms to identify and handle outliers that may skew the data, ensuring a more accurate dataset for analysis.
### 4. **Data Enrichment**
- **Supplementary Data Sources**: Integrate additional data sources (e.g., market trends, material specifications) to provide context and enhance the quality of the primary data collected.
- **Feature Engineering**: Develop new features from existing data that can improve model performance and provide deeper insights into material characteristics.
### 5. **Training and Skill Development**
- **Staff Training**: Provide training for staff on the importance of data quality and the proper methods for data collection and handling.
- **Cross-Functional Teams**: Create interdisciplinary teams that include data scientists, engineers, and operators to collaboratively improve data collection and analysis processes.
### 6. **Implementing Data Governance Practices**
- **Data Management Policies**: Establish clear policies regarding data ownership, access, and usage to ensure accountability and improve data integrity.
- **Data Audits**: Conduct regular audits of data collection and storage practices to identify areas for improvement and ensure compliance with data quality standards.
### 7. **Utilizing Advanced Technologies**
- **IoT and Smart Sensors**: Implement Internet of Things (IoT) devices and smart sensors that can provide real-time data and improve the accuracy of readings.
- **Machine Learning for Data Quality**: Use machine learning algorithms to continuously assess and improve data quality by identifying patterns and anomalies in the data.
### 8. **Feedback Loops**
- **Continuous Improvement**: Create a feedback mechanism where insights from data analysis are used to refine data collection methods and processes.
- **User Feedback**: Encourage feedback from operators and data users to identify practical challenges and areas where data collection can be improved.
### 9. **Collaboration with External Partners**
- **Industry Partnerships**: Collaborate with industry experts, academic institutions, and technology providers to share best practices and gain insights into improving data quality.
- **Benchmarking**: Compare data quality metrics with industry standards to identify gaps and areas for improvement.
### Conclusion
By employing these strategies, recycling facilities can significantly enhance the quality of their data, leading to better decision-making and improved efficiency in operations. High-quality data is essential for the successful application of machine learning algorithms, ultimately contributing to more effective recycling processes and outcomes.
6mm Rose Gold Classic Tungsten Ring - Carbide CUSTOM MADE Engraved Men Women | Wedding bands
### 1. **Standardized Data Collection Processes**
- **Consistent Protocols**: Establish standardized procedures for collecting data from sensors and equipment to ensure uniformity tungsten cube plated in gold data formats and types.
- **Automated Data Logging**: Implement automated systems for logging data to minimize human error and ensure consistent data capture.
### 2. **Regular Calibration of Equipment**
- **Sensor Calibration**: Regularly calibrate sensors and imaging systems to ensure accurate readings and measurements, reducing noise and inaccuracies in the data.
- **Maintenance Checks**: Schedule routine maintenance to ensure all equipment functions properly, minimizing data quality issues caused by malfunctioning devices.
### 3. **Data Cleaning and Preprocessing**
- **Noise Reduction**: Employ techniques to filter out noise and correct errors in the data using statistical methods or machine learning approaches.
- **Outlier Detection**: Implement algorithms to identify and handle outliers that may skew the data, ensuring a more accurate dataset for analysis.
### 4. **Data Enrichment**
- **Supplementary Data Sources**: Integrate additional data sources (e.g., market trends, material specifications) to provide context and enhance the quality of the primary data collected.
- **Feature Engineering**: Develop new features from existing data that can improve model performance and provide deeper insights into material characteristics.
### 5. **Training and Skill Development**
- **Staff Training**: Provide training for staff on the importance of data quality and the proper methods for data collection and handling.
- **Cross-Functional Teams**: Create interdisciplinary teams that include data scientists, engineers, and operators to collaboratively improve data collection and analysis processes.
### 6. **Implementing Data Governance Practices**
- **Data Management Policies**: Establish clear policies regarding data ownership, access, and usage to ensure accountability and improve data integrity.
- **Data Audits**: Conduct regular audits of data collection and storage practices to identify areas for improvement and ensure compliance with data quality standards.
### 7. **Utilizing Advanced Technologies**
- **IoT and Smart Sensors**: Implement Internet of Things (IoT) devices and smart sensors that can provide real-time data and improve the accuracy of readings.
- **Machine Learning for Data Quality**: Use machine learning algorithms to continuously assess and improve data quality by identifying patterns and anomalies in the data.
### 8. **Feedback Loops**
- **Continuous Improvement**: Create a feedback mechanism where insights from data analysis are used to refine data collection methods and processes.
- **User Feedback**: Encourage feedback from operators and data users to identify practical challenges and areas where data collection can be improved.
### 9. **Collaboration with External Partners**
- **Industry Partnerships**: Collaborate with industry experts, academic institutions, and technology providers to share best practices and gain insights into improving data quality.
- **Benchmarking**: Compare data quality metrics with industry standards to identify gaps and areas for improvement.
### Conclusion
By employing these strategies, recycling facilities can significantly enhance the quality of their data, leading to better decision-making and improved efficiency in operations. High-quality data is essential for the successful application of machine learning algorithms, ultimately contributing to more effective recycling processes and outcomes.
Black Classic Domed 8mm Classic Tungsten Ring - Carbide CUSTOM MADE Engraved Men Women | Wedding bands
Improving data quality in recycling facilities is crucial for the successful implementation of machine learning algorithms and overall operational efficiency. Here are several strategies that can be employed:
### 1. **Standardized Data Collection Processes**
- **Consistent Protocols**: Establish standardized procedures for collecting data from sensors and equipment to ensure uniformity in data formats and types.
- **Automated Data Logging**: Implement automated systems for logging data to minimize human error and ensure consistent data capture.
### 2. **Regular Calibration of Equipment**
- **Sensor Calibration**: Regularly calibrate sensors and imaging systems to ensure accurate readings and measurements, reducing noise and inaccuracies in the data.
- **Maintenance Checks**: Schedule routine maintenance to ensure all equipment functions properly, minimizing data quality issues caused by malfunctioning devices.
### 3. **Data Cleaning and Preprocessing**
- **Noise Reduction**: Employ techniques to filter out noise and correct errors in the data using statistical methods or machine learning approaches.
- **Outlier Detection**: Implement algorithms to identify and handle outliers that may skew the data, ensuring a more accurate dataset for analysis.
### 4. **Data Enrichment**
- **Supplementary Data Sources**: Integrate additional data sources (e.g., market trends, material specifications) to provide context and enhance the quality of the primary data collected.
- **Feature Engineering**: Develop new features from existing data that can improve model performance and provide deeper insights into material characteristics.
### 5. **Training and Skill Development**
- **Staff Training**: Provide training for staff on the importance of data quality and the proper methods for data collection and handling.
- **Cross-Functional Teams**: Create interdisciplinary teams that include data scientists, engineers, and operators to collaboratively improve data collection and analysis processes.
### 6. **Implementing Data Governance Practices**
- **Data Management Policies**: Establish clear policies regarding data ownership, access, and usage to ensure accountability and improve data integrity.
- **Data Audits**: Conduct regular audits of data collection and storage practices to identify areas for improvement and ensure compliance with data quality standards.
### 7. **Utilizing Advanced Technologies**
- **IoT and Smart Sensors**: Implement Internet of Things (IoT) devices and smart sensors that can provide real-time data and improve the accuracy of readings.
- **Machine Learning for Data Quality**: Use machine learning algorithms to continuously assess and improve data quality by identifying patterns and anomalies in the data.
### 8. **Feedback Loops**
- **Continuous Improvement**: Create a feedback mechanism where insights from data analysis are used to refine data collection methods and processes.
- **User Feedback**: Encourage feedback from operators and data users to identify practical challenges and areas where data collection can be improved.
### 9. **Collaboration with External Partners**
- **Industry Partnerships**: Collaborate with industry experts, academic institutions, and technology providers to share best practices and gain insights into improving data quality.
- **Benchmarking**: Compare data quality metrics with industry standards to identify gaps and areas for improvement.
### Conclusion
By employing these strategies, recycling facilities can significantly enhance the quality of their data, leading to better decision-making and improved efficiency in operations. High-quality data is essential for the successful application of machine learning algorithms, ultimately contributing to more effective recycling processes and outcomes.
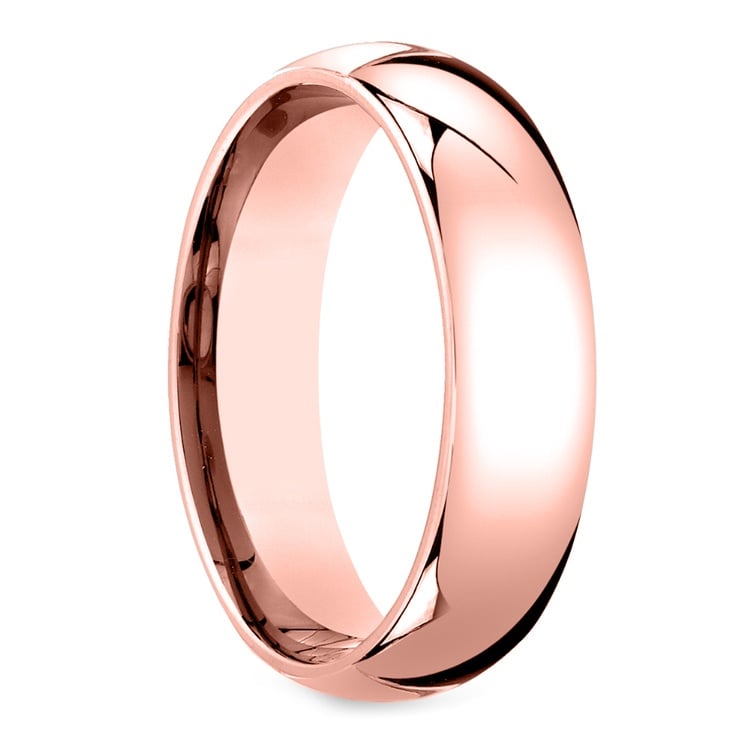