Implementing machine learning algorithms in a recycling facility, particularly for tungsten carbide recycling, presents several challenges. Here are some key issues that may arise:
### 1. **Data Quality and Availability**
- **Insufficient Data**: Effective machine learning models require large amounts of high-quality data for training. In recycling facilities, obtaining sufficient labeled data (e.g., identifying different materials) can be difficult.
- **Noise and Inconsistency**: Data collected from sensors and imaging systems may contain noise or inconsistencies, which can hinder the performance of algorithms.
### 2. **Complexity of Material Identification**
- **Varied Material Composition**: Tungsten carbide is often mixed with other metals or materials, making it challenging to classify accurately.
- **Diverse Shapes and Sizes**: The physical characteristics of materials can vary widely, complicating the sorting process and requiring robust algorithms capable of handling this variability.
### 3. **Integration with Existing Systems**
- **Legacy Equipment**: Many recycling facilities may use outdated or incompatible systems, making it difficult to integrate new machine learning solutions.
- **Operational Disruption**: Implementing new technologies may require adjustments to existing workflows, potentially causing temporary disruptions in operations.
### 4. **Computational Resources**
- **Processing Power**: Some machine learning algorithms, especially deep learning models, require significant computational resources for training and inference. Facilities may need to invest in hardware and software infrastructure.
- **Real-Time Processing**: Sorting materials often requires real-time analysis, which can be challenging if the computational capabilities are not sufficient.
### 5. **Expertise and Training**
- **Lack of Skilled Personnel**: Implementing machine learning solutions requires expertise in data science and machine learning, which may be lacking in recycling facilities.
- **Training Requirements**: Staff may need training to operate and maintain new systems, which can be time-consuming and costly.
### 6. **Maintenance and Updates**
- **Model Drift**: Over time, the characteristics of incoming materials may change, leading to "model drift," where existing models become less effective. Regular updates and retraining are necessary to maintain accuracy.
- **Ongoing Maintenance**: Machine learning systems require continuous monitoring and maintenance to ensure optimal performance, which can add to operational overhead.
### 7. **Cost Considerations**
- **High Initial Investment**: The upfront costs associated with implementing machine learning technologies (e.g., hardware, software, training) can be significant.
- **Uncertain ROI**: The return on investment may not be immediately clear, making it challenging to justify the costs to stakeholders.
### 8. **Regulatory and Compliance Issues**
- **Adherence to Regulations**: Recycling facilities must comply with various environmental and safety regulations, which may complicate the implementation of new technologies.
- **Data Privacy Concerns**: If data collection involves sensitive information, there may be concerns regarding data privacy and security.
### Conclusion
While machine learning offers significant potential to enhance the efficiency and accuracy of material sorting in tungsten carbide recycling, these challenges must be addressed for successful implementation. Overcoming these obstacles often requires careful planning, investment in training and infrastructure, and ongoing support to ensure that machine learning systems operate effectively within the recycling facility's environment.
Gold Mercury Tungsten Carbide Ring 6mm - Carbide CUSTOM MADE Engraved Men Women | Wedding bands
Implementing machine learning algorithms in a recycling facility, particularly for tungsten carbide recycling, presents several challenges. Here are some key issues that may arise:
### 1. **Data Quality and Availability**
- **Insufficient Data**: Effective machine learning models require large amounts of high-quality data for training. In recycling facilities, obtaining sufficient labeled data (e.g., identifying different materials) can be difficult.
- **Noise and Inconsistency**: Data collected from sensors and imaging systems may contain noise or inconsistencies, which can hinder the performance of algorithms.
### 2. **Complexity of Material Identification**
- **Varied Material Composition**: grooved black tungsten ring with beveled edge carbide is often mixed with other metals or materials, making it challenging to classify accurately.
- **Diverse Shapes and Sizes**: The physical characteristics of materials can vary widely, complicating the sorting process and requiring robust algorithms capable of handling this variability.
### 3. **Integration with Existing Systems**
- **Legacy Equipment**: Many recycling facilities may use outdated or incompatible systems, making it difficult to integrate new machine learning solutions.
- **Operational Disruption**: Implementing new technologies may require adjustments to existing workflows, potentially causing temporary disruptions in operations.
### 4. **Computational Resources**
- **Processing Power**: Some machine learning algorithms, especially deep learning models, require significant computational resources for training and inference. Facilities may need to invest in hardware and software infrastructure.
- **Real-Time Processing**: Sorting materials often requires real-time analysis, which can be challenging if the computational capabilities are not sufficient.
### 5. **Expertise and Training**
- **Lack of Skilled Personnel**: Implementing machine learning solutions requires expertise in data science and machine learning, which may be lacking in recycling facilities.
- **Training Requirements**: Staff may need training to operate and maintain new systems, men's tungsten carbide brushed center rose gold cross grooved ring which can be time-consuming and costly.
### 6. **Maintenance and Updates**
- **Model Drift**: Over time, the characteristics of incoming materials may change, leading to "model drift," where existing models become less effective. Regular updates and retraining are necessary to maintain accuracy.
- **Ongoing Maintenance**: Machine learning systems require continuous monitoring and maintenance to ensure optimal performance, which can add to operational overhead.
### 7. **Cost Considerations**
- **High Initial Investment**: The upfront costs associated with implementing machine learning technologies (e.g., hardware, software, training) can be significant.
- **Uncertain ROI**: The return on investment may not be immediately clear, making it challenging to justify the costs to stakeholders.
### 8. **Regulatory and Compliance Issues**
- **Adherence to Regulations**: Recycling facilities must comply with various environmental and safety regulations, which may complicate the implementation of new technologies.
- **Data Privacy Concerns**: If data collection involves sensitive information, there may be concerns regarding data privacy and security.
### Conclusion
While machine learning offers significant potential to enhance the efficiency and accuracy of material sorting in tungsten carbide recycling, these challenges must be addressed for successful implementation. Overcoming these obstacles often requires careful planning, investment in training and infrastructure, and ongoing support to ensure that machine learning systems operate effectively within the recycling facility's environment.
Astrea Tungsten Ring 4mm - Carbide CUSTOM MADE Engraved Men Women | Wedding bands
### 1. **Data Quality and Availability**
- **Insufficient Data**: Effective machine learning models require large amounts of high-quality data for training. In recycling facilities, obtaining sufficient labeled data (e.g., identifying different materials) can be difficult.
- **Noise and Inconsistency**: Data collected from sensors and imaging systems may contain noise or inconsistencies, which can hinder the performance of algorithms.
### 2. **Complexity of Material Identification**
- **Varied Material Composition**: Tungsten carbide is often mixed with other metals or materials, making it challenging to classify accurately.
- **Diverse Shapes and Sizes**: The physical characteristics of materials can vary widely, complicating the sorting process and requiring robust algorithms capable of handling this variability.
### 3. **Integration with Existing Systems**
- **Legacy Equipment**: Many recycling facilities may use outdated or incompatible systems, making it difficult to integrate new machine learning solutions.
- **Operational Disruption**: Implementing new technologies may require adjustments to existing workflows, potentially causing temporary disruptions in operations.
### 4. **Computational Resources**
- **Processing Power**: Some machine learning algorithms, especially deep learning models, require significant computational resources for training and inference. Facilities may need to invest in hardware and software infrastructure.
- **Real-Time Processing**: Sorting materials often requires real-time analysis, which can be challenging if the computational capabilities are not sufficient.
### 5. **Expertise and Training**
- **Lack of Skilled Personnel**: Implementing machine learning solutions requires expertise in data science and machine learning, which may be lacking in recycling facilities.
- **Training Requirements**: Staff may need training to operate and maintain new systems, which can be time-consuming and costly.
### 6. **Maintenance and Updates**
- **Model Drift**: Over time, the characteristics of incoming materials may change, leading to "model drift," where existing models become less effective. Regular updates and retraining are necessary to maintain accuracy.
- **Ongoing Maintenance**: Machine learning systems require continuous monitoring and maintenance to ensure optimal performance, which can add to operational overhead.
### 7. **Cost Considerations**
- **High Initial Investment**: The upfront costs associated with implementing machine learning technologies (e.g., hardware, software, training) can be significant.
- **Uncertain ROI**: The return on investment may not be immediately clear, making it challenging to justify the costs to stakeholders.
### 8. **Regulatory and Compliance Issues**
- **Adherence to Regulations**: Recycling facilities must comply with various environmental and safety regulations, which may complicate the implementation of new technologies.
- **Data Privacy Concerns**: If data collection involves sensitive information, there may be concerns regarding data privacy and security.
### Conclusion
While machine learning offers significant potential to enhance the efficiency and accuracy of material sorting in tungsten carbide recycling, these challenges must be addressed for successful implementation. Overcoming these obstacles often requires careful planning, investment in training and infrastructure, and ongoing support to ensure that machine learning systems operate effectively within the recycling facility's environment.
Gold Mercury Tungsten Carbide Ring 6mm - Carbide CUSTOM MADE Engraved Men Women | Wedding bands
Implementing machine learning algorithms in a recycling facility, particularly for tungsten carbide recycling, presents several challenges. Here are some key issues that may arise:
### 1. **Data Quality and Availability**
- **Insufficient Data**: Effective machine learning models require large amounts of high-quality data for training. In recycling facilities, obtaining sufficient labeled data (e.g., identifying different materials) can be difficult.
- **Noise and Inconsistency**: Data collected from sensors and imaging systems may contain noise or inconsistencies, which can hinder the performance of algorithms.
### 2. **Complexity of Material Identification**
- **Varied Material Composition**: grooved black tungsten ring with beveled edge carbide is often mixed with other metals or materials, making it challenging to classify accurately.
- **Diverse Shapes and Sizes**: The physical characteristics of materials can vary widely, complicating the sorting process and requiring robust algorithms capable of handling this variability.
### 3. **Integration with Existing Systems**
- **Legacy Equipment**: Many recycling facilities may use outdated or incompatible systems, making it difficult to integrate new machine learning solutions.
- **Operational Disruption**: Implementing new technologies may require adjustments to existing workflows, potentially causing temporary disruptions in operations.
### 4. **Computational Resources**
- **Processing Power**: Some machine learning algorithms, especially deep learning models, require significant computational resources for training and inference. Facilities may need to invest in hardware and software infrastructure.
- **Real-Time Processing**: Sorting materials often requires real-time analysis, which can be challenging if the computational capabilities are not sufficient.
### 5. **Expertise and Training**
- **Lack of Skilled Personnel**: Implementing machine learning solutions requires expertise in data science and machine learning, which may be lacking in recycling facilities.
- **Training Requirements**: Staff may need training to operate and maintain new systems, men's tungsten carbide brushed center rose gold cross grooved ring which can be time-consuming and costly.
### 6. **Maintenance and Updates**
- **Model Drift**: Over time, the characteristics of incoming materials may change, leading to "model drift," where existing models become less effective. Regular updates and retraining are necessary to maintain accuracy.
- **Ongoing Maintenance**: Machine learning systems require continuous monitoring and maintenance to ensure optimal performance, which can add to operational overhead.
### 7. **Cost Considerations**
- **High Initial Investment**: The upfront costs associated with implementing machine learning technologies (e.g., hardware, software, training) can be significant.
- **Uncertain ROI**: The return on investment may not be immediately clear, making it challenging to justify the costs to stakeholders.
### 8. **Regulatory and Compliance Issues**
- **Adherence to Regulations**: Recycling facilities must comply with various environmental and safety regulations, which may complicate the implementation of new technologies.
- **Data Privacy Concerns**: If data collection involves sensitive information, there may be concerns regarding data privacy and security.
### Conclusion
While machine learning offers significant potential to enhance the efficiency and accuracy of material sorting in tungsten carbide recycling, these challenges must be addressed for successful implementation. Overcoming these obstacles often requires careful planning, investment in training and infrastructure, and ongoing support to ensure that machine learning systems operate effectively within the recycling facility's environment.
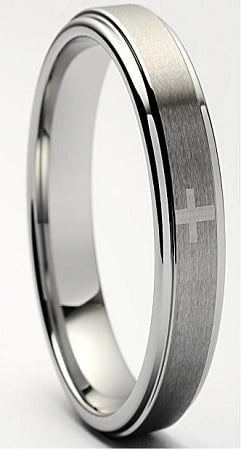